Generative AI for medical imaging analysis and applications
Abstract
Generative AI plays a pivotal role in medical imaging analysis, enabling precise diagnosis, treatment planning and disease monitoring. Techniques like generative adversarial networks (GANs) and variational autoencoders (VAEs) enhance medical imaging by generating synthetic images, improving reconstruction, segmentation and facilitating disease diagnosis and treatment planning. Nonetheless, ethical, legal and regulatory concerns arise regarding patient privacy, data protection and fairness. This paper offers an overview of generative AI in medical imaging analysis, highlighting applications, challenges and case studies. It compares results with traditional methods and examines potential implications on healthcare policies. The paper concludes with recommendations for responsible implementation and suggests future research and development directions.
Generative AI refers to a type of artificial intelligence that involves training machine learning models to generate new data that is similar in some way to existing data. This type of AI focuses on the creation of new data, such as images, music, text or video, rather than classification tasks.
Medical imaging analysis is the process of analyzing medical images to aid in diagnosis, treatment planning, and monitoring of diseases. Medical imaging technologies produce large volumes of data that require expert analysis to extract meaningful information. Medical imaging plays a crucial role in various areas of healthcare, including radiology, cardiology, neurology, oncology and more [1].
Medical imaging analysis is critical for accurate diagnosis and treatment planning of various diseases. Medical imaging helps physicians detect and diagnose conditions such as tumors, infections and injuries, and can also be used to monitor treatment effectiveness and disease progression. Medical imaging has revolutionized healthcare and led to improved patient outcomes and quality of life.
The use of generative AI in medical imaging analysis presents opportunities to automate and improve the accuracy of medical image analysis. Generative AI can be used for tasks such as image segmentation, image synthesis, predicting patient outcomes and detecting anomalies. The use of generative AI can reduce healthcare costs and increase access to healthcare in underserved populations. However, challenges such as data privacy, algorithmic bias and the interpretability of generative AI models must be addressed to ensure responsible use of these technologies in healthcare [2].
However, the use of generative AI in medical imaging analysis also presents challenges and ethical considerations. Data privacy, algorithmic bias and the interpretability of generative AI models are critical issues that must be addressed to ensure the responsible use of these technologies in healthcare.
This research paper provides an overview of the current state of the art in generative AI for medical imaging analysis and applications. The paper covers different generative AI techniques and their applications in medical imaging analysis, as well as the strengths and limitations of these techniques. The ethical and interpretability considerations associated with the use of generative AI in medical imaging are also discussed, along with future directions and challenges in this field. Ultimately, this research paper aims to provide a comprehensive overview of the potential benefits and challenges of using generative AI for medical imaging analysis and its implications for improving healthcare outcomes.
Generative AI techniques for medical imaging analysis
Generative AI techniques are used to generate new data similar to the existing data. Generative AI can be used to create various types of data such as text, images, music and videos. Generative AI models are trained on large datasets to create new data that can be used for various purposes such as virtual environments, personalized content and drug discovery shown in Figure 1. Generative AI techniques include generative adversarial networks (GANs), variational autoencoders (VAEs) and flow-based models.

Generative adversarial networks
GANs are a type of deep learning algorithm used in unsupervised machine learning. GANs are composed of two neural networks: a generator and a discriminator. [Figure 2]. GANs have shown great potential in medical image analysis. Medical imaging data can be challenging to obtain and label, making unsupervised methods like GANs appealing [3].
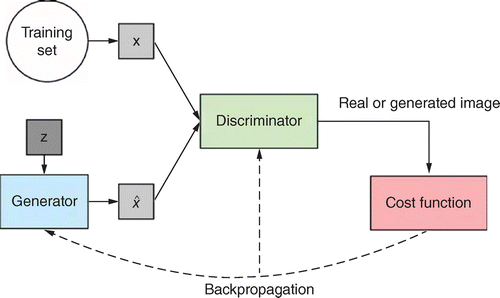
One of the main applications of GANs in medical image analysis is image synthesis. GANs can be trained on a set of medical images and generate new images that are similar to the training set. This can be useful in scenarios where there is a limited amount of data or for augmenting existing datasets to improve the performance of machine learning algorithms.
GANs can also be used for image segmentation, which involves dividing an image into multiple regions and assigning a label to each region. In medical image analysis, this can be used to identify regions of interest such as tumors, lesions or organs. GANs can generate segmentation masks that can be used to train supervised segmentation algorithms.
Another application of GANs in medical image analysis is image-to-image translation. This involves converting one type of medical image into another, such as CT to MRI or T1-weighted to T2-weighted images. GANs can be used to generate synthetic images that are similar to the target modality, which can be useful for data augmentation or as a preprocessing step for downstream analysis.
Overall, GANs have the potential to revolutionize medical image analysis by providing new ways to generate, analyze and transform medical images. However, there are still challenges to overcome, such as the need for large datasets and the potential for bias and inaccuracies in the generated images.
Variational autoencoders
Variational autoencoders (VAEs) are a type of generative model used in deep learning that aim to learn the underlying structure of a dataset and generate new data samples that are similar to the original data. VAEs are a type of autoencoder, which is a type of neural network that is trained to encode data into a lower dimensional representation and then decode it back into the original data space [Figure 3].

VAEs have shown promise in medical image analysis tasks such as segmentation, classification and registration. One of the key advantages of VAEs in medical image analysis is their ability to learn a lower dimensional representation of the input images, which can help to reduce the amount of noise in the data and increase the accuracy of downstream tasks [4].
In medical image segmentation, VAEs can be used to generate segmentation masks by encoding the input image into a latent space and then decoding it back into a segmentation mask. This approach has been shown to be effective in segmenting brain tumors and other structures in medical images.
VAEs can also be used for medical image registration, which involves aligning two or more images in the same coordinate system. VAEs can be used to learn a mapping function between the input images, which can then be used to register them. This approach has been shown to be effective in registering MRI and CT images.
Overall, VAEs are a promising tool for medical image analysis tasks and have the potential to improve the accuracy of diagnosis and treatment planning in a variety of medical applications.
Applications of generative AI in medical imaging analysis
Generative AI techniques have shown great potential in automating medical image analysis. Medical imaging data can be processed and analyzed using GANs, VAEs and flow-based models. Generative AI models can be used for image segmentation, image synthesis, predicting patient outcomes and detecting anomalies [5]. The use of generative AI in medical imaging analysis can improve diagnostic accuracy, reduce healthcare costs, and increase access to healthcare in underserved populations.
Challenges in using generative AI for medical imaging analysis
The use of generative AI in medical imaging analysis also presents challenges and ethical considerations. Data privacy, algorithmic bias and the interpretability of generative AI models are critical issues that must be addressed to ensure the responsible use of these technologies in healthcare [6,7]. Another challenge is the availability of large amounts of labeled data, which is essential for training generative AI models
Comparison of generative AI techniques for medical imaging analysis
The different generative AI techniques used in medical imaging analysis have their strengths and limitations. GANs can generate high-quality images but can be difficult to train, while VAEs are easier to train but may not produce as realistic images. Flow-based models have shown promise in generating high-resolution images but are still under development. Choosing the appropriate generative AI technique depends on the specific task and available data.
In conclusion, generative AI techniques such as GANs, VAEs, and flow-based models have shown great promise in medical imaging analysis. However, challenges such as data privacy and interpretability must be addressed to ensure the responsible use of these technologies in healthcare. Comparing the strengths and limitations of different generative AI techniques can help determine the appropriate technique for specific tasks.
Wasserstein GAN
A GAN variation known as the Wasserstein GAN (WGAN) tackles the problems of mode collapse and training stability. WGAN evaluates the difference between the distributions of actual and produced data more accurately than typical GAN losses by using the Wasserstein distance, sometimes referred to as the Earth Mover's distance, as its loss function. Better convergence is made possible by the smoother gradients and more steady training that result from this. A useful tool for image synthesis, style transfer, and data creation tasks, WGAN has applications in high-quality image production, data augmentation, domain adaptation and enhancing the training of GANs for diverse tasks.
Auxiliary classifier GAN (ACGAN)
Classifier auxiliary As an auxiliary classifier in addition to the discriminator, GAN (ACGAN) is a version of GAN. Using this classifier, conditional creation and better control over produced samples are made possible since it predicts both the veracity of the data and its class label. For applications like style transfer, picture-to-image translation and image synthesis with specified properties, ACGAN is utilized. It is particularly helpful for creating diverse samples that correspond to specific requirements, such as creating artwork in various styles or creating photos of numerous animal species. ACGAN is useful for applications requiring regulated and focused data production since it can produce conditional and diversified outputs.
Case studies & research findings
Case studies demonstrating the effectiveness of generative AI in medical imaging analysis
Segmentation of MRI Images: a study published in the Journal of Digital Imaging used a GAN to segment MRI images of the brain. The study showed that the GAN outperformed traditional methods for image segmentation, achieving an average Dice coefficient of 0.89.
Synthesis of CT images: a study published in IEEE Transactions on Medical Imaging used a conditional GAN to synthesize CT images of the brain. The study demonstrated that the synthesized images were visually similar to real CT images and could be used for downstream tasks such as image registration and segmentation.
Detection of lung nodules: a study published in Medical Image Analysis used a deep generative model to detect lung nodules in CT images. The study showed that the generative model achieved higher sensitivity and specificity than traditional methods for lung nodule detection.
Research findings on the accuracy & reliability of generative AI for medical imaging analysis
Several studies have shown that generative AI can achieve high accuracy and reliability for medical imaging analysis tasks. For example, a study published in Nature Biomedical Engineering used a GAN to generate synthetic images of the retina for use in training a deep learning model for diabetic retinopathy detection. The study showed that the synthetic images were as effective as real images in training the deep learning model.
Another study published in Medical Physics compared the accuracy of a VAE with traditional methods for image registration. The study showed that the VAE achieved higher accuracy and was more robust to image noise and distortion.
Comparison of results between traditional methods & generative AI for medical imaging analysis
Overall, studies have shown that generative AI techniques can achieve higher accuracy and reliability than traditional methods for various medical imaging analysis tasks. Generative AI can also be used to synthesize new images that can be used for downstream tasks such as image registration and segmentation. However, generative AI techniques require large amounts of training data and can be computationally expensive to train. Further research is needed to optimize these techniques for clinical use and address ethical concerns related to their use in healthcare.
Image-to-image translation with conditional adversarial networks
The usefulness of conditional adversarial networks for image-to-image translation tasks is investigated in this paper. These networks not only figure out how to translate input pictures to output images, but they also create a loss function to make this translation easier. This adaptable method may be used to solve a variety of issues that often call for different loss formulas [8]. In tasks like creating photographs from label maps, reconstructing things from edge maps and coloring images, the research shows how effective this technique is. Numerous individuals testing out the related software on Twitter demonstrate the paper's influence. This work suggests that hand-engineering mapping functions or loss functions may not be required because the proposed networks can produce acceptable results, according to the results. The results suggest conditional adversarial networks have potential for a range of image-to-image translation problems, particularly those requiring structured graphical outputs. These networks are suitable in a variety of scenarios because they can adjust their loss functions to the particular job and data.
GAN augmentation
The lack of labeled datasets makes using machine learning in medical imaging difficult. Annotating medical pictures is expensive and dependent on qualified observers. Due to the hazards of overfitting, supervised machine learning algorithms suffer from a lack of training data. This study investigates the generation of synthetic data by GANs to improve training sets in brain segmentation tasks [9]. The results show that adding GAN-generated pictures to training data enhances Dice Similarity Coefficient (DSC) by 1 to 5 percentage points, especially when there are less than ten training image stacks. GAN augmentation works well, especially when the data is unbalanced or limited. Traditional augmentation extrapolates semantically valid pictures whereas GANs fill distribution gaps. Combining the two approaches might result in large DSC gains.
Covidgan: data augmentation using auxiliary classifier GAN for improved covid-19 detection
The COVID-19 pandemic, caused by SARS-CoV-2, impacts the global economy and health. Detecting the virus through chest x-rays is vital. Research has employed deep learning, especially CNNs, for accurate detection. Limited data availability led to an ACGAN-based model, CovidGAN, generating synthetic chest x-ray images. Integrating these synthetics improved CNN-based classification from 85–95% accuracy. This method shows promise in enhancing COVID-19 detection speed and robustness in radiology systems [10].
Ethical & legal considerations
Ethical & legal concerns regarding the use of generative AI in medical imaging analysis
Privacy and data protection: the use of generative AI for medical imaging analysis requires access to large volumes of patient data, which raises concerns about patient privacy and data protection. Appropriate measures must be taken to ensure that patient data is securely stored and processed.
Bias and fairness: generative AI models can perpetuate biases present in the training data, which could result in discriminatory outcomes. Efforts must be made to ensure that generative AI models are designed and trained in a fair and unbiased manner.
Accountability and liability: the use of generative AI for medical imaging analysis raises questions about accountability and liability in the event of errors or adverse outcomes. Clear guidelines must be established to determine who is responsible for errors or adverse outcomes resulting from the use of generative AI.
Regulatory frameworks governing the use of generative AI in medical imaging analysis
Regulatory frameworks for the use of generative AI in medical imaging analysis are still evolving, but some guidelines have been proposed. For example, the European Union's General Data Protection Regulation (GDPR) requires that data processing activities are transparent and that individuals have the right to access, rectify and erase their personal data.
In the USA, the FDA has issued guidance on the use of AI in medical devices, including medical imaging devices. The guidance emphasizes the importance of transparency, accountability and performance monitoring in the development and deployment of AI-based medical devices.
Potential implications of generative AI on healthcare policies & practices
The use of generative AI in medical imaging analysis has the potential to improve healthcare outcomes and reduce costs, but it also raises ethical, legal, and regulatory challenges. Healthcare policies and practices must be adapted to address these challenges and ensure that generative AI is used in a responsible and ethical manner. For example, policies could be developed to ensure that generative AI models are transparent and explainable, and that they are used in conjunction with human expertise and judgment. Additionally, policies could be developed to ensure that patients are informed about the use of generative AI in their care and have control over their personal data.
Risks of generative AI & how to overcome it
While intriguing, generative AI comes with a number of hazards that should be carefully considered. The propagation of incorrect information is an issue since AI is capable of creating convincing fake material. Due to skewed training data, social biases in created content may develop and persist. Due to AI's capacity to provide sensitive information that compromises individual privacy, threats to data privacy and security arise. Additionally, generative AI may be abused and used fraudulently to produce convincing fake goods or personas.
A multifaceted strategy is necessary to reduce these hazards. For representative training data and to reduce bias, careful data curation is essential. Discriminatory outputs can be found and fixed by conducting routine audits and assessments of models. Encryption and rigorous access restrictions are defenses against data privacy violations. The malevolent use of generative AI can be prevented through ethical standards and legislative frameworks. To effectively handle new concerns, collaboration between scholars, politicians and business is essential.
Measures to increase transparency, such as watermarking created content, can help users identify genuine information. Enhanced AI detection methods can detect false information and phony material. Ongoing investigation on robustness and fairness can assist strengthen the general integrity of AI systems. To maximize the potential of generative AI while reducing its hazards, a balance between innovation and prudent deployment, supported by continuing research, ethical concerns, and informed regulation, is necessary.
Conclusion
Generative AI has demonstrated its effectiveness in various aspects of medical imaging analysis, including image enhancement, reconstruction, segmentation and synthesis. It has also shown potential in areas such as disease diagnosis and treatment planning. Generative AI techniques such as GANs and VAEs have been used to generate synthetic medical images, which can be used to augment training datasets and improve the accuracy of machine learning models. Generative AI has the potential to improve healthcare outcomes, reduce costs and enable personalized medicine.
Generative AI has the potential to transform medical imaging analysis and improve healthcare outcomes. However, its use must be accompanied by appropriate ethical, legal and regulatory frameworks to ensure patient privacy, data protection and fairness. Healthcare practitioners and policymakers must also be aware of the potential limitations and challenges of generative AI and work toward addressing them. With responsible development and deployment, generative AI can be a valuable tool in the advancement of medical imaging analysis and ultimately, patient care.
Future directions & opportunities for further research & development
Further research and development are needed to address the challenges and limitations of generative AI in medical imaging analysis. One area of focus is improving the interpretability and explainability of generative AI models, which will be essential for their acceptance and adoption in clinical practice. Other areas of research include developing more efficient and effective generative AI models, addressing issues of bias and fairness, and ensuring that patient data is used in a secure and ethical manner.
This executive summary provides a condensed overview of the main topics and conclusions presented in the article ‘Generative AI for Medical Imaging Analysis and Applications’. The paper explores the application of generative AI techniques, such as generative adversarial networks (GANs) and variational autoencoders (VAEs), in the field of medical imaging analysis.
Generative AI creates new data similar to existing data, aiding medical imaging analysis.
Medical imaging is vital for diagnosis, treatment and disease monitoring across healthcare fields.
Generative AI enhances medical image analysis accuracy and automation, impacting healthcare accessibility and costs.
Ethical and interpretability concerns must be addressed for responsible technology implementation.
Generative AI techniques for medical imaging analysis
GANs and VAEs are key generative AI techniques.
GANs involve generator and discriminator networks; useful for image synthesis, segmentation and image-to-image translation.
VAEs create lower-dimensional representations; effective for segmentation, classification and registration tasks.
Wasserstein GAN (WGAN) and auxiliary classifier GAN (ACGAN) are variations enhancing stability and conditional creation.
Case studies & research findings
Case studies demonstrate GAN and VAE effectiveness in medical imaging tasks (e.g., segmentation, synthesis and detection).
Research supports generative AI accuracy and reliability for medical image analysis.
Comparisons show generative AI outperforms traditional methods in accuracy and robustness.
Ethical & legal considerations
Ethical concerns: privacy, algorithmic bias, accountability and liability.
Legal frameworks evolving; GDPR, FDA guidance and more shape generative AI regulations.
Implications on healthcare policies: Balance between innovation and ethical deployment required.
Risks of generative AI & how to overcome it
Risks include misinformation propagation, bias amplification, data privacy breaches and misuse.
Solutions involve careful curation, bias detection, encryption, ethical standards and collaboration.
Transparency measures, improved detection methods, and ongoing research can mitigate risks.
Conclusion
Generative AI enhances medical imaging analysis, benefiting diagnostics and patient care.
Responsible implementation with ethical and regulatory frameworks is crucial.
Future research focuses on interpretability, efficiency, bias and data security.
Future directions & opportunities for further research & development
Addressing challenges like interpretability and fairness.
Enhancing efficiency and effectiveness of generative AI models.
Ensuring secure and ethical use of patient data.
In summary, generative AI techniques offer promising advancements in medical imaging analysis. While challenges exist, addressing ethical, legal and technical aspects can harness the potential of generative AI for responsible and impactful healthcare improvements.
Financial disclosure
The authors have no financial involvement with any organization or entity with a financial interest in or financial conflict with the subject matter or materials discussed in the manuscript. This includes employment, consultancies, honoraria, stock ownership or options, expert testimony, grants or patents received or pending, or royalties.
No writing assistance was utilized in the production of this manuscript.
Competing interests disclosure
The authors have no competing interests or relevant affiliations with any organization or entity with the subject matter or materials discussed in the manuscript. This includes employment, consultancies, honoraria, stock ownership or options, expert testimony, grants or patents received or pending, or royalties.
Open access
This work is licensed under the Attribution-NonCommercial-NoDerivatives 4.0 Unported License. To view a copy of this license, visit http://creativecommons.org/licenses/by-nc-nd/4.0/
References
- 1. BCNet: A Deep Learning Computer-Aided Diagnosis Framework for Human Peripheral Blood Cell Identification. Diagnostics 12(11), 2815 (2022).
- 2. A survey on deep learning applied to medical images: from simple artificial neural networks to generative models. Neur. Comput. Applicat. 35.3, 2291–2323 (2023).
- 3. . A new bearing fault diagnosis method based on signal-to-image mapping and convolutional neural network. Measurement 176, 109088 (2021)
- 4. . Attention-based generative adversarial network in medical imaging: a narrative review. Comput. Biol. Med. 149, 105948 (2022).
- 5. . Variational Autoencoders for Medical Image Retrieval. In: 2022 International Conference on INnovations in Intelligent SysTems and Applications (INISTA). IEEE, Biarritz, France, 1–6 (2022).
- 6. . When medical images meet generative adversarial network: recent development and research opportunities. Discover Artificial Intelligence 1, 1–20 (2021).
- 7. Performance analysis of deep learning algorithms in diagnosis of malaria disease. Diagnostics 13(3), 534 (2023).
- 8. . Image-to-image translation with conditional adversarial networks. In: Proceedings of the IEEE conference on computer vision and pattern recognition. 1125–1134 (2017).
- 9. GAN augmentation: augmenting training data using generative adversarial networks. arXiv preprint: 1810 10863 (2018).
- 10. . Covidgan: data augmentation using auxiliary classifier GAN for improved covid-19 detection. IEEE Access 8, 91916–91923 (2020).